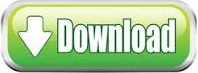
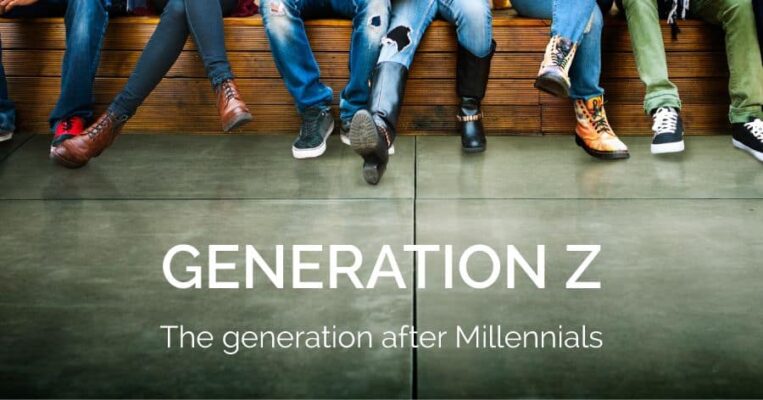
This approach outperforms or provides comparable results with the best traditional autofocus method on small and large image patches respectively. To address these issues, we present a deep learning-based autofocus framework that can estimate the position of the objective-lens focal plane relative to the light-sheet, based on two defocused images. Yet, autofocus techniques are slow since they require capturing a stack of images and tend to fail in the presence of spherical aberrations that dominate volume imaging. To address this issue, autofocus methods are commonly used to refocus the focal plane of the objective-lens on the light-sheet. However, spatial heterogeneity in the refractive index of the specimen often results in violation of this requirement when imaging deep in the tissue. A fundamental requirement for LSFM is a seamless overlap of the light-sheet that excites a selective plane in the specimen, with the focal plane of the objective lens. Light-sheet fluorescence microscopy (LSFM) is a minimally invasive and high throughput imaging technique ideal for capturing large volumes of tissue with sub-cellular resolution. Overall, our study provides a framework that can correct the angle of the light-sheet and improve the overall image quality in high-resolution LSFM 3D image acquisition. The angular estimation was based on calculating the defocus level on a pixel level within the image using two defocused images. The illumination beam was then corrected using a pair of galvo scanners, and the correction significantly improved the image quality across the entire field-of-view. To address these issues, we tested a deep learning-based approach to estimate the angular error of the illumination beam relative to the detection focal plane.
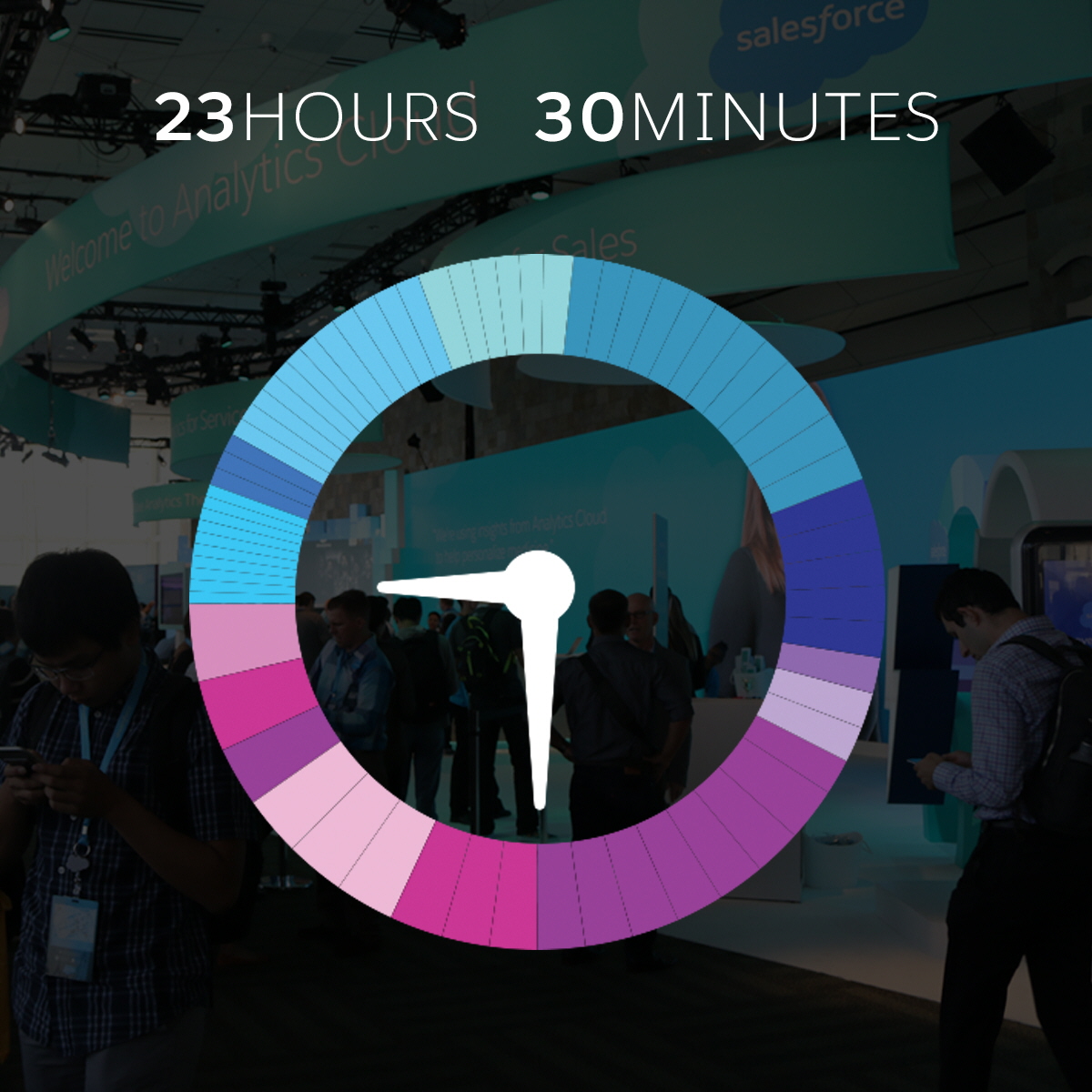
This refraction can cause extensive blur and non-uniform image quality over the imaged field-of-view. However, spatial heterogeneity within the sample, curved specimen boundaries, and mismatch of refractive index between tissues and immersion media can refract the well-aligned illumination beam. As such, the image quality, especially at high resolution, largely depends on the degree of overlap between the detection focal plane and the illuminating beam.

LSFM has a unique configuration, in which the illumination and detection paths are separated and perpendicular to each other. LSFM is routinely used in life sciences for live cell imaging and for capturing large volumes of cleared tissues.

Light-sheet fluorescence microscopy (LSFM) is a high-speed imaging technique that provides optical sectioning with reduced photodamage. The results which we obtained suggest that the proposed autofocus model based on multi-ROI window can be used effectively in scanning of cervical cell images. Validation on another dataset CC proves its robustness, comparison with the recent studies shows its practical performance. Comprehensive analysis denotes that the proposed autofocus algorithm achieves the accuracy of 93.7% and an average focusing time (sec/mm²) of 11.89. Multi-ROI (Region of interest) focus window consisting of image processing, selective search and BP (Back Propagation) neural network is used for the autofocus. The proposed approach applies multiple-median filter and histogram equalization for the image denoising. Aiming at this problem, a novel focus method based on multi regions of interest window is presented. Due to the different focal lengths of cervical areas in smears, existing focusing approaches often result in blurry images of lymphocytes and epithelial cells, which are the keys for the cervical cancer detection. Autofocus methods play crucial roles in optical systems, which closely relate to the collected image quality.
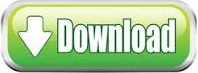